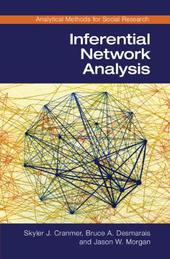
|
Inferential Network Analysis
Hardback
Main Details
Description
This unique textbook provides an introduction to statistical inference with network data. The authors present a self-contained derivation and mathematical formulation of methods, review examples, and real-world applications, as well as provide data and code in the R environment that can be customised. Inferential network analysis transcends fields, and examples from across the social sciences are discussed (from management to electoral politics), which can be adapted and applied to a panorama of research. From scholars to undergraduates, spanning the social, mathematical, computational and physical sciences, readers will be introduced to inferential network models and their extensions. The exponential random graph model and latent space network model are paid particular attention and, fundamentally, the reader is given the tools to independently conduct their own analyses.
Author Biography
Skyler J. Cranmer is the Carter Phillips and Sue Henry Professor of Political Science at The Ohio State University. Bruce A. Desmarais is the DeGrandis-McCourtney Early Career Professor in Political Science at Penn State University. Jason William Morgan is the Vice President for Behavioural Intelligence: Aware, and visiting scholar in Political Science at The Ohio State University.
Reviews'The family of exponential random graph models have advanced with a number of extensions in recent years, many of them developed by the present authors. Encapsulating these advances with other methods of inferential analysis in a single reference that combines essential theory with hands-on examples makes this book a must-have for network modeling practitioners who want to use these powerful tools.' Peter Mucha, UNC Chapel Hill
|