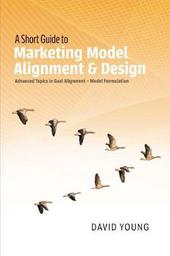
|
A Short Guide to Marketing Model Alignment & Design: Advanced Topics in Goal Alignment - Model Formulation
Paperback / softback
Main Details
Title |
A Short Guide to Marketing Model Alignment & Design: Advanced Topics in Goal Alignment - Model Formulation
|
Authors and Contributors |
By (author) David Young
|
Physical Properties |
Format:Paperback / softback | Pages:66 | Dimensions(mm): Height 228,Width 152 |
|
Category/Genre | Probability and statistics |
ISBN/Barcode |
9781543912593
|
Audience | |
|
Publishing Details |
Publisher |
BookBaby
|
Imprint |
BookBaby
|
Publication Date |
16 November 2017 |
Publication Country |
United States
|
Description
How this book is different from other Marketing Modeling books and what you can get from itThis book integrates Marketing and Statistical concepts and is written for Marketing Modelers, Model Users, and Purchasers of marketing models. Most books on Marketing Modeling are slightly modified statistical texts. If you're looking for a book that talks about the "logic of marketing" and the "design of statistical models" in an integrated way to increase model accuracy and improve business profits, then this book was written for you. Marketing Models are neither just Statistics nor just Marketing, but a synthesis of the information sources creating a cohesive predictive system, and the broader you cast your net for useful information the better your models will be.Nevertheless, anyone who's worked around Marketing Models at all will have heard people talk about modifying models for "statistical reasons" or modifying them for "business reasons" as though the two sets of criteria are from Mars and Venus, respectively. In this book, I try to help readers develop a deeper understanding of the reasoning behind both sets of rules to put themselves in a better position to weigh the value of all evidence and define the most applicable business goals for their models to address. And after defining those goals, design the best models for achieving them. Too often, modeling objectives are defined quickly and without much thought. In many cases, standard statistical techniques are pulled from the "tool box" and applied without aim. Oftentimes the models resulting from that process are reasonable, although typically not optimal. Other times they're clearly lacking, however the business users (and sometimes competent modelers) are fooled by the facade of science surrounding the modeling process. Hence, criticism is often deflected and the models can live on, even if their efficacy has become dubious. If you'd like to better understand: *how to define dependent variables to maximize business goals;*how business logic should influence your model design; *when lower R-Squared statistics can represent better models; *how much information you can reasonably expect from your data;*how to safely work with imperfect data that may offer partial information but that shouldn't be naively relied upon, and; *ultimately how to create models offering superior business value ... then read on.
Author Biography
David Young has 25 years of experience in Marketing Modeling applied to Marketing Mix Modeling, Choice Modeling, Market Segmentation, Propensity Models, etc. He currently works as a Senior Group Director at Neustar in McLean, VA managing a team of 10 modelers whom optimize about 2 billion dollars of annual advertising dollars across multiple, often multinational clients. A native of the USA, he also lived in Madrid, Spain for ten years and speaks Spanish passably well. He's an invited speaker at various Data Science venues.
|