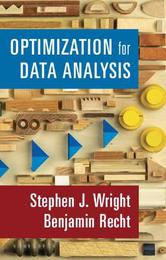
|
Optimization for Data Analysis
Hardback
Main Details
Title |
Optimization for Data Analysis
|
Authors and Contributors |
By (author) Stephen J. Wright
|
|
By (author) Benjamin Recht
|
Physical Properties |
Format:Hardback | Pages:238 | Dimensions(mm): Height 235,Width 156 |
|
Category/Genre | Maths for engineers Data capture and analysis |
ISBN/Barcode |
9781316518984
|
Classifications | Dewey:519.6 |
---|
Audience | Professional & Vocational | Tertiary Education (US: College) | |
Illustrations |
Worked examples or Exercises; Worked examples or Exercises
|
|
Publishing Details |
Publisher |
Cambridge University Press
|
Imprint |
Cambridge University Press
|
Publication Date |
21 April 2022 |
Publication Country |
United Kingdom
|
Description
Optimization techniques are at the core of data science, including data analysis and machine learning. An understanding of basic optimization techniques and their fundamental properties provides important grounding for students, researchers, and practitioners in these areas. This text covers the fundamentals of optimization algorithms in a compact, self-contained way, focusing on the techniques most relevant to data science. An introductory chapter demonstrates that many standard problems in data science can be formulated as optimization problems. Next, many fundamental methods in optimization are described and analyzed, including: gradient and accelerated gradient methods for unconstrained optimization of smooth (especially convex) functions; the stochastic gradient method, a workhorse algorithm in machine learning; the coordinate descent approach; several key algorithms for constrained optimization problems; algorithms for minimizing nonsmooth functions arising in data science; foundations of the analysis of nonsmooth functions and optimization duality; and the back-propagation approach, relevant to neural networks.
Author Biography
Stephen J. Wright holds the George B. Dantzig Professorship, the Sheldon Lubar Chair, and the Amar and Balinder Sohi Professorship of Computer Sciences at the University of Wisconsin-Madison and a Discovery Fellow in the Wisconsin Institute for Discovery. He works in computational optimization and its applications to data science and many other areas of science and engineering. He is a Fellow of SIAM, and recipient of the 2014 W. R. G. Baker Award from IEEE for most outstanding paper, the 2020 Khachiyan Prize by the INFORMS Optimization Society for lifetime achievements in optimization, and the 2020 NeurIPS Test of Time Award. Professor Wright is the author and co-author of widely used textbooks and reference books in optimization including Primal Dual Interior-Point Methods (1987) and Numerical Optimization (2006). Benjamin Recht is an Associate Professor in the Department of Electrical Engineering and Computer Sciences at the University of California, Berkeley. His research group studies how to make machine learning systems more robust to interactions with a dynamic and uncertain world by using mathematical tools from optimization, statistics, and dynamical systems. Professor Recht is the recipient of a Presidential Early Career Award for Scientists and Engineers, an Alfred P. Sloan Research Fellowship, the 2012 SIAM/MOS Lagrange Prize in Continuous Optimization, the 2014 Jamon Prize, the 2015 William O. Baker Award for Initiatives in Research, and the 2017 and 2020 NeurIPS Test of Time Awards.
Reviews'This delightful compact tome gives the reader all the results they should have in their pocket to contribute to optimization and statistical learning. With the clean, elegant derivations of many of the foundational optimization methods underlying modern large-scale data analysis, everyone from students just getting started to researchers knowing this book inside and out will be well-positioned for both using the algorithms and developing new ones for machine learning, optimization, and statistics.' John C. Duchi, Stanford University 'Optimization algorithms play a vital role in the rapidly evolving field of machine learning, as well as in signal processing, statistics and control. Numerical optimization is a vast field, however, and a student wishing to learn the methods required in the world of data science could easily get lost in the literature. This book does a superb job of presenting the most important algorithms, providing both their mathematical foundations and lucid motivations for their development. Written by two of the foremost experts in the field, this book gently guides a reader without prior knowledge of optimization towards the methods and concepts that are central in modern data science applications.' Jorge Nocedal, Northwestern University 'This timely introductory book gives a rigorous view of continuous optimization techniques which are being used in machine learning. It is an excellent resource for those who are interested in understanding the mathematical concepts behind commonly used machine learning techniques.' Shai Shalev-Shwartz, Hebrew University of Jerusalem 'This textbook is a much-needed exposition of optimization techniques, presented with conciseness and precision, with emphasis on topics most relevant for data science and machine learning applications. I imagine that this book will be immensely popular in university courses across the globe, and become a standard reference used by researchers in the area.' Amitabh Basu, Johns Hopkins University
|